Conclusion
Neural networks are suitable for predicting time series mainly because of learning only from examples, without any need to add additional information that can bring more confusion than prediction effect. Neural networks are able to generalize and are resistant to noise. On the other hand, it is generally not possible to determine exactly what a neural network learned and it is also hard to estimate possible prediction error.
However, neural networks were often successfully used for predicting time series (see references). They are ideal especially when we do not have any other description of the observed series.
References
Note: the original text and applet was written in Czech, that's why the references are mainly Czech ones. You can use any search engine to find other references or applications.
[1] Dostál. D: Předpovídání časových řad, 1997, diplomová práce, FEL ČVUT
[2] Doubek J: Předpovídání časové řady, semestrální práce, FEL ČVUT
[3] Pattreson D.: Artificial Neural networks - Theory and Applications, Prentice Hall, 1996
[4] Polívka: Předpovídání časových řad, 1998, diplomová práce, FEL ČVUT
[5] Šnorek M., Jiřina M.: Neuronové sítě a neuropočítače, Vydavatelsví ČVUT, 1996
About
This text and the illustration applet was created as a semestral work at the Czech Technical University in Prague by Marek Obitko.
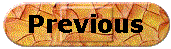
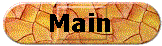
(c) Marek Obitko, 1999 - Terms of use